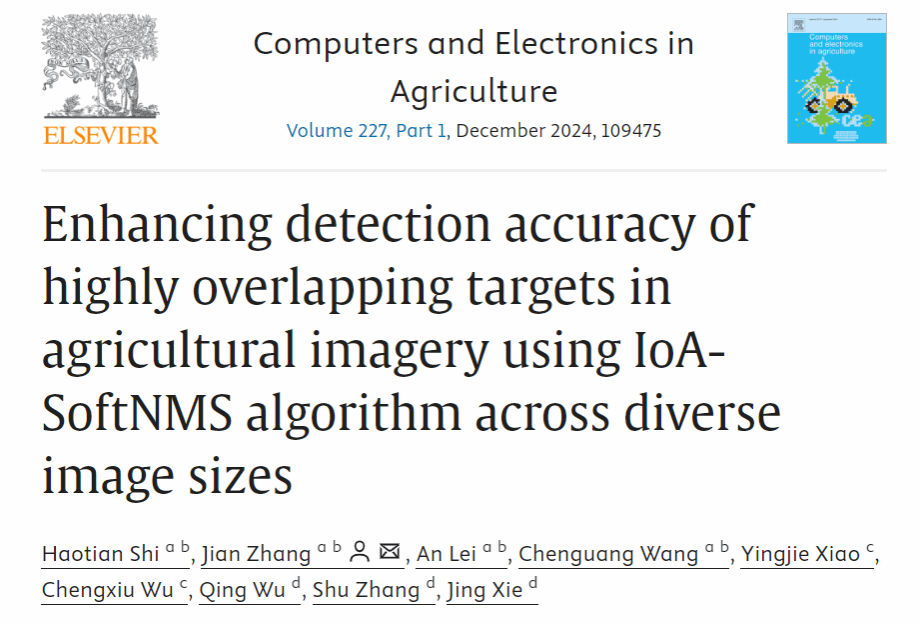
Abstract / 摘要
Object detection is crucial for agricultural monitoring and agriculture automation, yet conventional non-maximum suppression (NMS) algorithms often struggle in agricultural scenes with complex backgrounds and highly overlapping targets, especially in intersection over union (IoU) threshold setting and abnormal non-maximum removal. This study proposed the soft non-maximum suppression based on intersection over area (IoA-SoftNMS) algorithm, an advanced NMS algorithm designed to enhance detection accuracy in such challenging agricultural environments. IoA-SoftNMS employs intersection over area (IoA) to quantify overlap and integrate SoftNMS to boost overall detection precision and effectiveness. Validation experiments demonstrated that IoA-SoftNMS significantly increased detection accuracy, improving mean average precision (mAP) by 0.7 % to 3.5 % across various object detection models, without necessitating model retraining. Importantly, these enhancements barely affect the detection time. IoA-SoftNMS exhibited robust adaptability in detecting large images, especially when integrated with the slicing-aided hyper inference (SAHI) technique, where it notably improved detection accuracy during the slicing process. Application of IoA-SoftNMS elevates detection accuracy in medium (1000 × 3300 pixels) and large (2500 × 3400 pixels) images from 93 % to 96.9 % and 90.8 % to 95.3 %, respectively. These findings underscored the effectiveness of the proposed IoA-SoftNMS algorithm in improving object detection accuracy in agricultural settings and its potential to alleviate challenges posed by large image sizes, offering avenues for enhancing agricultural production precision and intelligence.